Paper Reading:Generalist World Model Pre-Training for Efficient Reinforcement Learning
本文最后更新于 2025年3月6日 下午
arxiv: https://arxiv.org/pdf/2502.19544v1
time:2025.2.26 ### Paper Reading:Generalist World Model Pre-Training for Efficient Reinforcement Learning
作者:

Summary: This paper specially consider reward-free and non-expert multi-embodiment offline data, and use a world model pre-Training WPT to guide the RL fine-tuning.
Emphasize the generalization of this method.
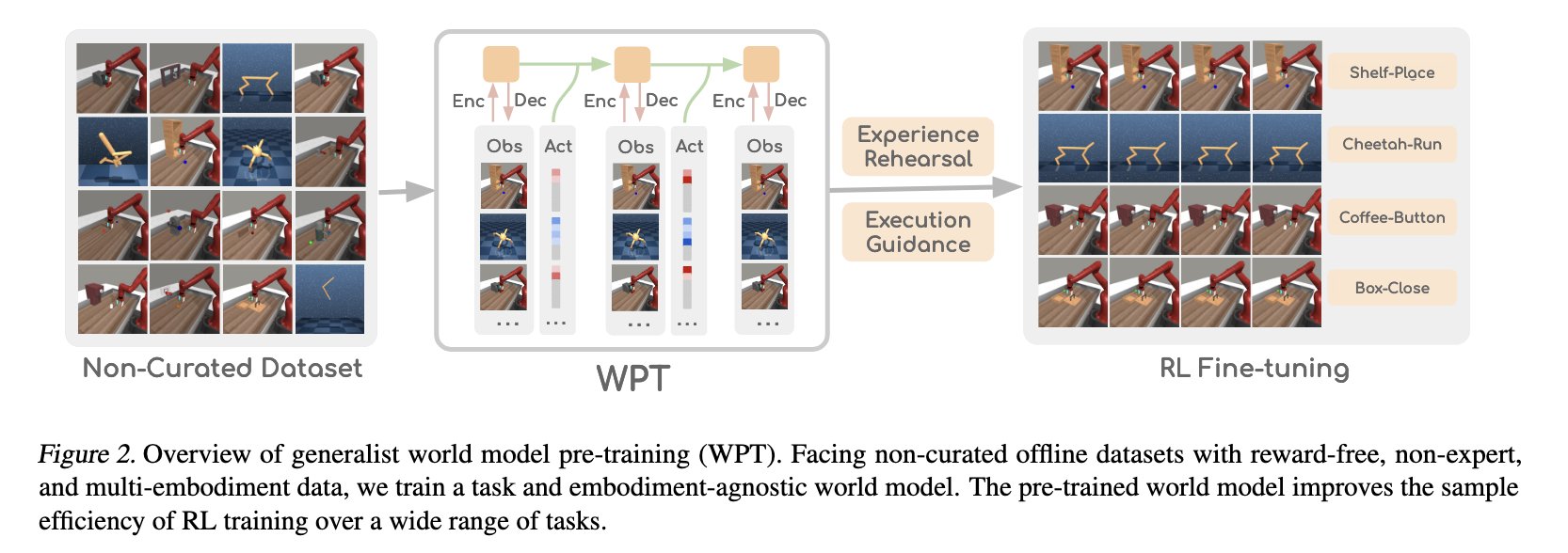
The experience rehearsal and execution guidance can generate useful initial state and guide the policy to collect the data closed to the offline data distribution in fine-tuning.
Result:
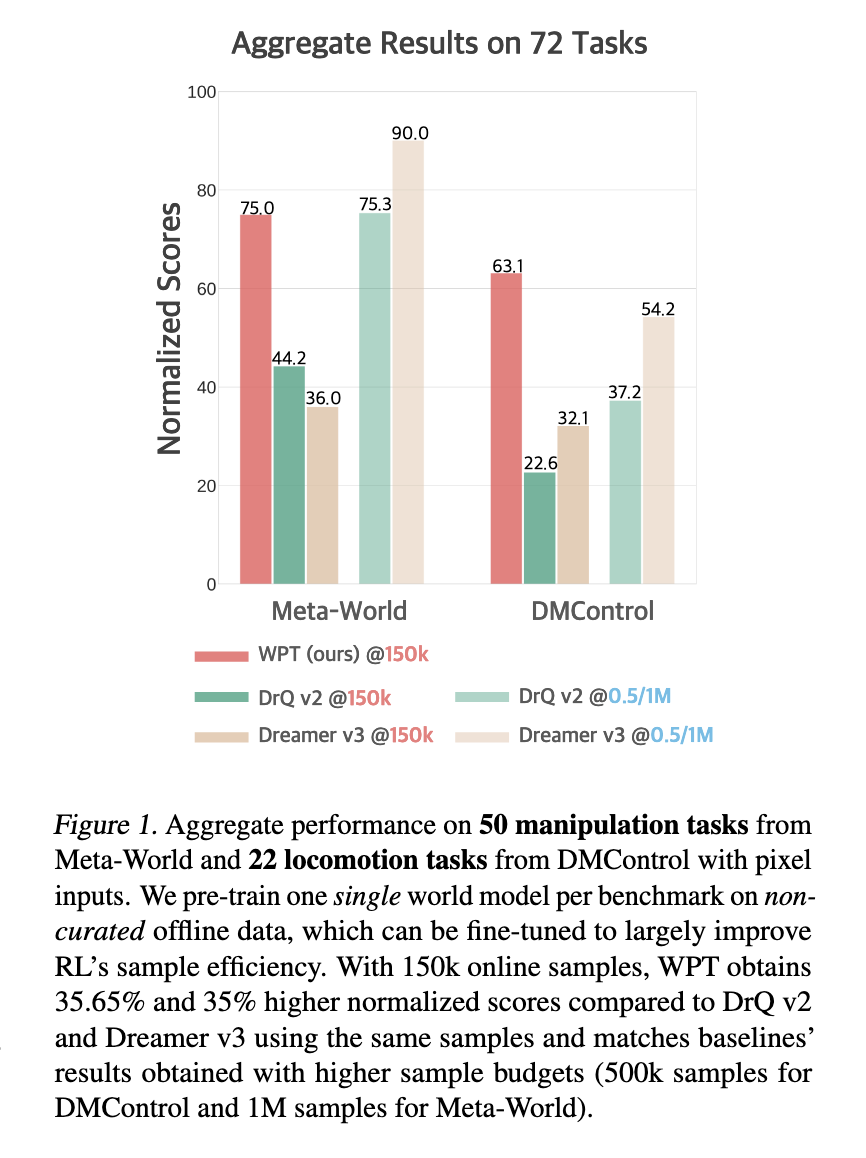
It makes the method converge faster, but its success rate is not higher than other offline learning methods.