Fine-Tuning Vision-Language-Action Models:Optimizing Speed and Success
本文最后更新于 2025年3月27日 中午
https://openvla-oft.github.io
Fine-Tuning Vision-Language-Action Models: Optimizing Speed and Success
Stanford
Summary:This paper explores how to fine-tune VLA model better, and they propose Open VLA OFT(Optimized Fine-Tuning) based on Open VLA which contains parallel decoding action chunking and a continuous action representation, and a simple L1 regression -base learning object to improve performance and inference efficiency.
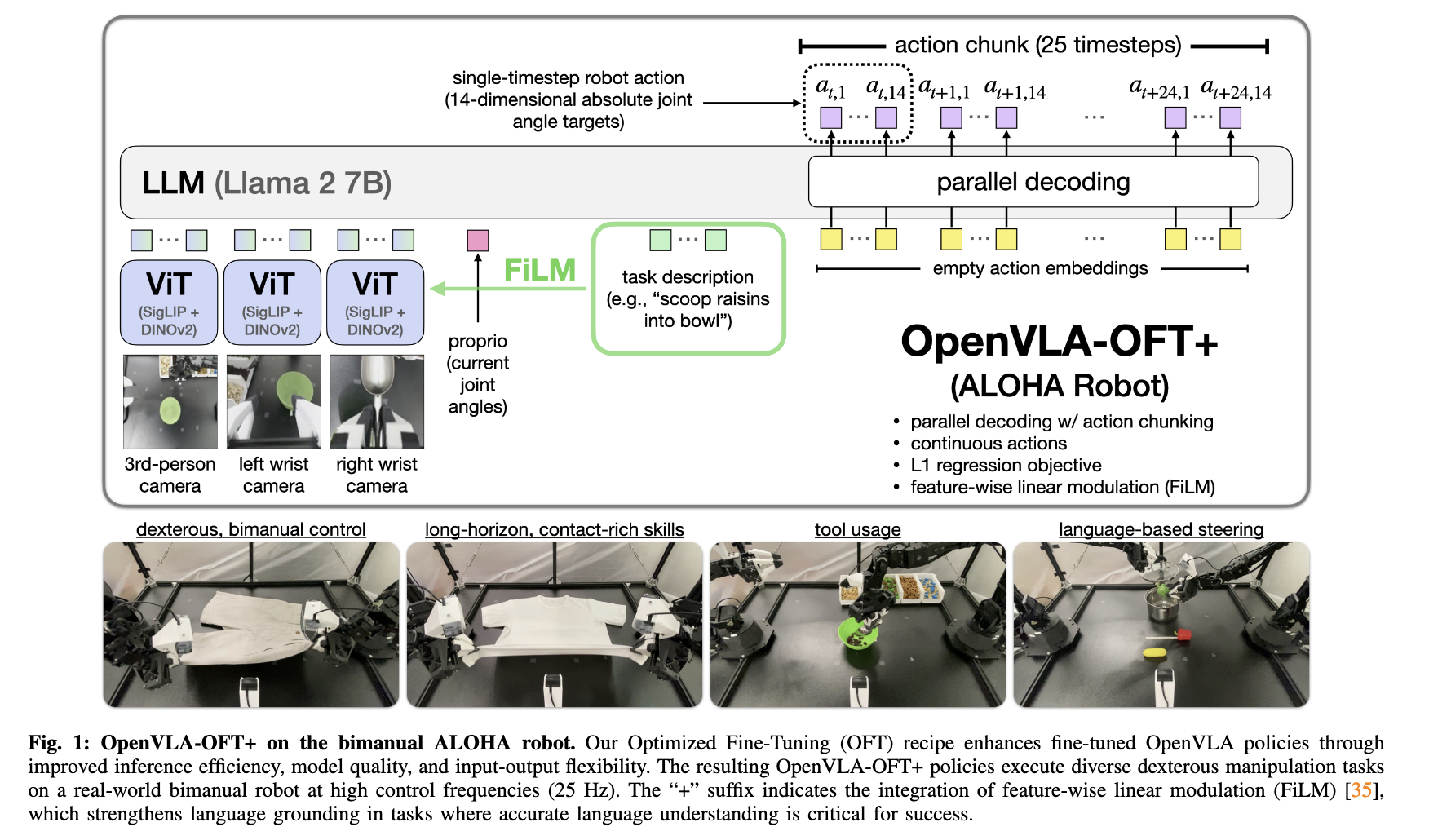
Parallel decoding:. We modify the model to receive empty action embeddings as input and replace the causal attention mask with bidirectional attention, allowing the decoder to predict all actions simultaneously.
Action policy: Discrete action tokens is not better than continuous action policy. The author think that may because the discrete action lose more details. L1 regression is simple and efficient just like my experimental experience.
FiLM: they also found that the policy struggle with language following due to spurious correlations in visual inputs, so they use FiLM(https://arxiv.org/abs/1709.07871)which infuse language embeddings into the visual representation so that the model can pay more attention to language inputs.
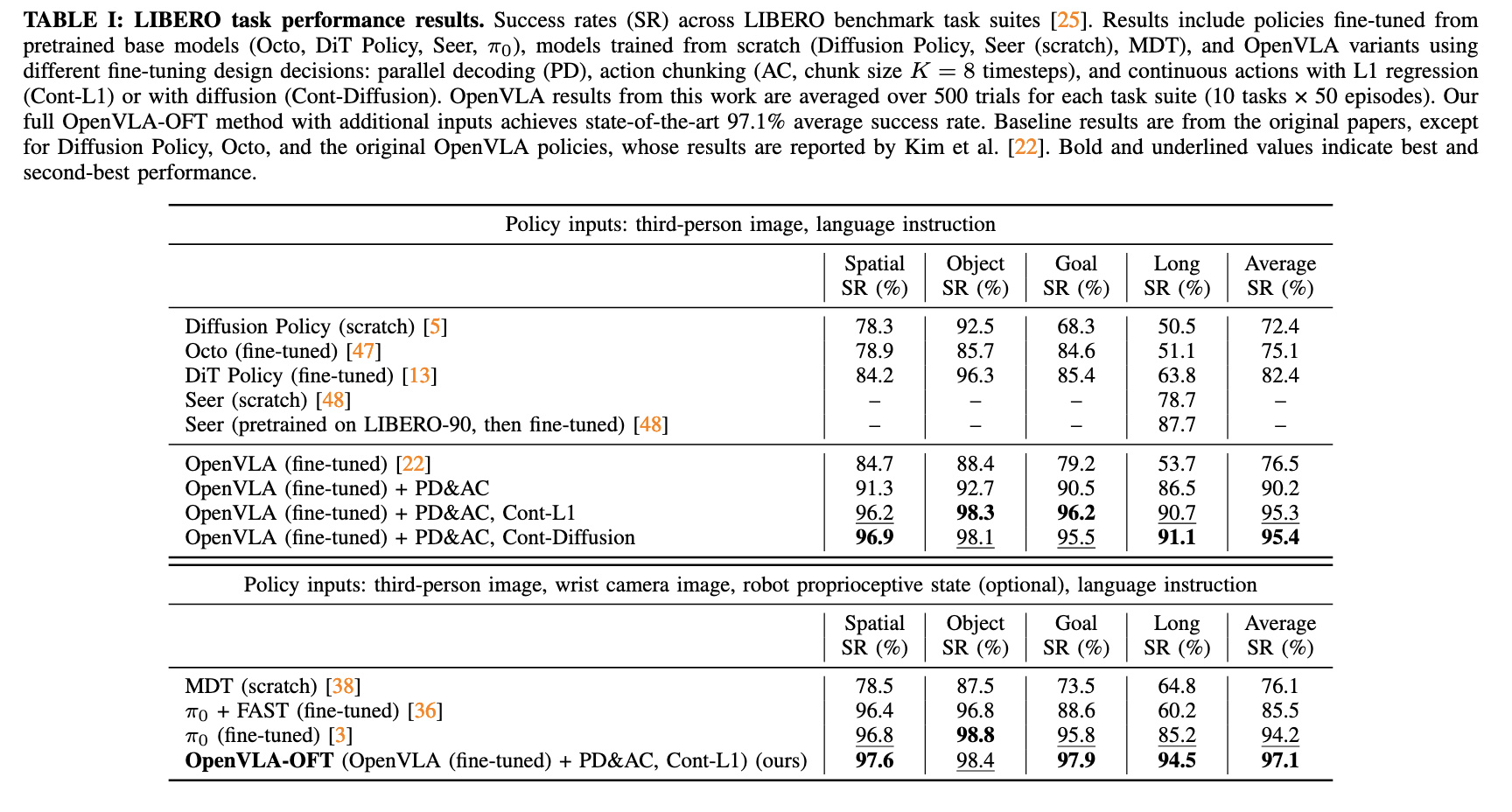
This result is consistent with my experiment findings: third image is enough to get a good performance.
Real robot experiment:
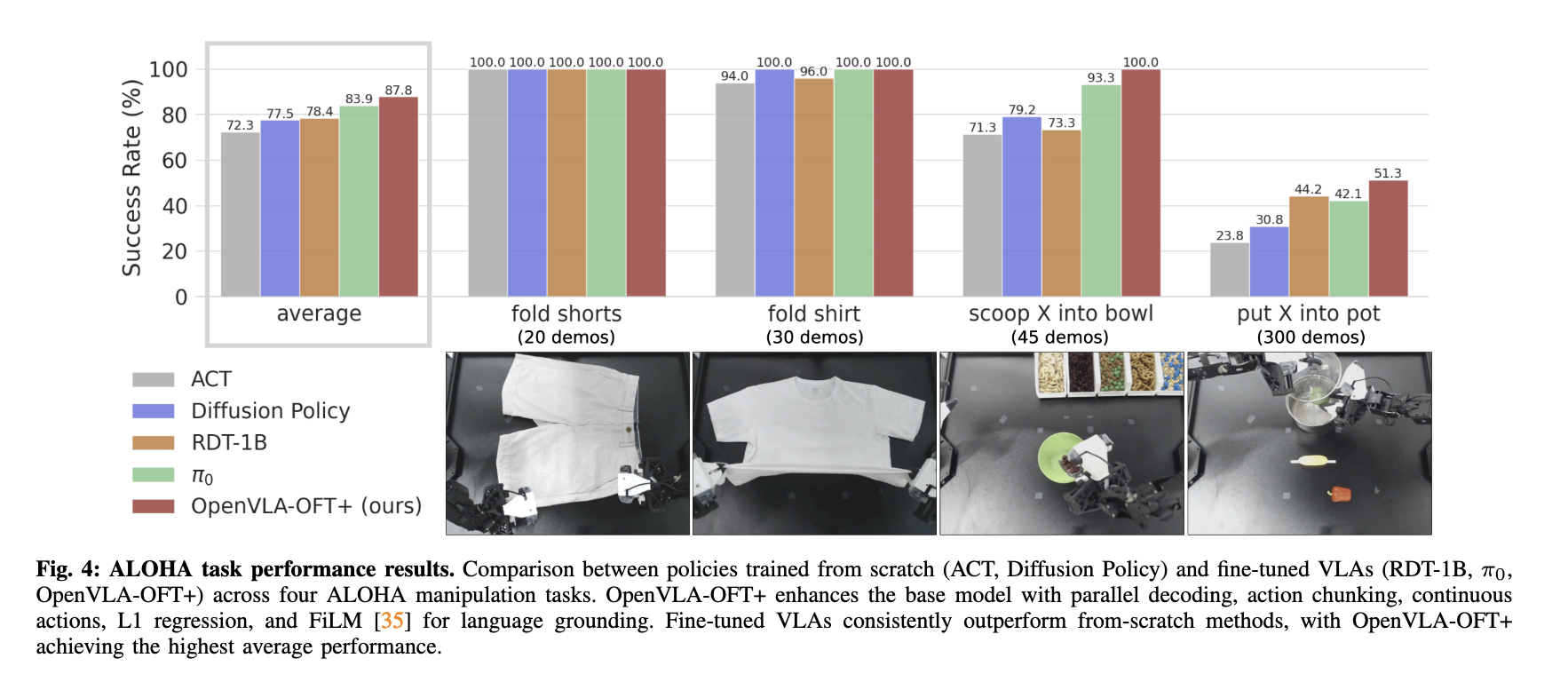